Members
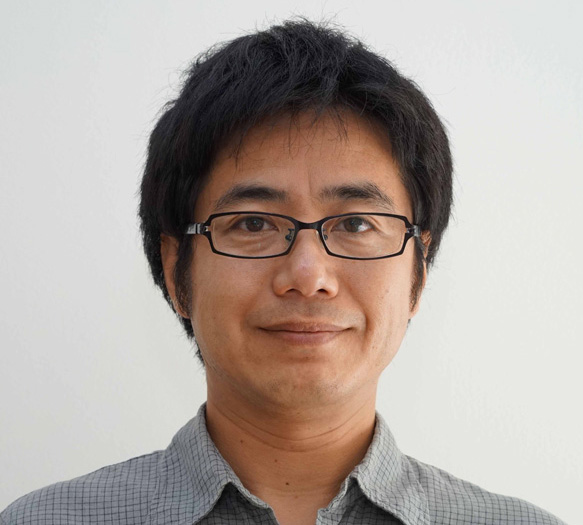
Junichi Chikazoe, MD, Ph.D.
Research Team Lead
M.D. and Ph.D. from the University of Tokyo Graduate School of Medicine. Post-doctoral work at the University of Toronto and Cornell University with Adam K. Anderson. After working as an associate professor at the Institute of Physiological Sciences, he joined Araya in 2021. His research focuses on creating AI with emotions. He is currently focusing on combining fMRI and deep learning to understand how value/valence emerges from sensory information. To see his latest research results, please click the above link or here.
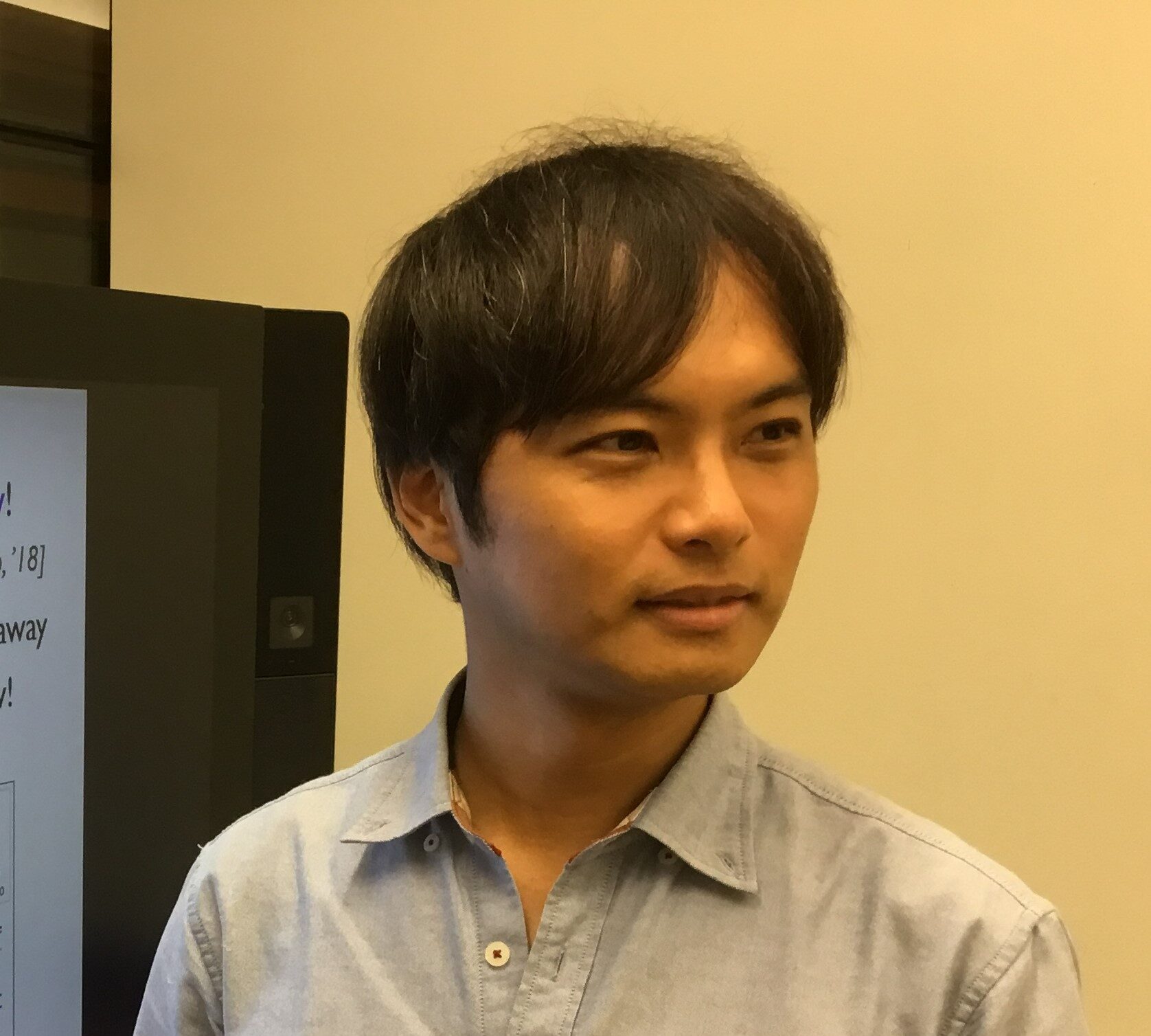
Shotaro Funai, Ph.D.
Chief Researcher
After receiving a Ph.D. from the University of Tokyo in 2010, he worked on physics research at several institutes such as High Energy Accelerator Research Organization (KEK). In 2016, he started researching machine learning, and in 2019, also started researching brain action through interdisciplinary collaborative research. He joined Araya in 2022. Based on his knowledge of physics, he is advancing data analysis of brain activity using machine learning.
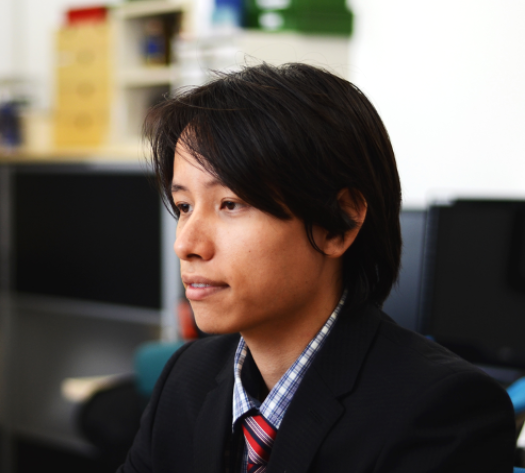
Pham Quang Trung, Ph.D.
Senior Researcher
Pham is a Senior Researcher at Araya. He received his Ph.D. in Mechanical Engineering at the Nagoya Institute of Technology. He did his postdoc with Junichi Chikazoe at National Institute for Physiological Science. He is interested in developing the computational models of brain and perception. He is a member of IEEE, SICE, and JNSS. His works can be found at here.
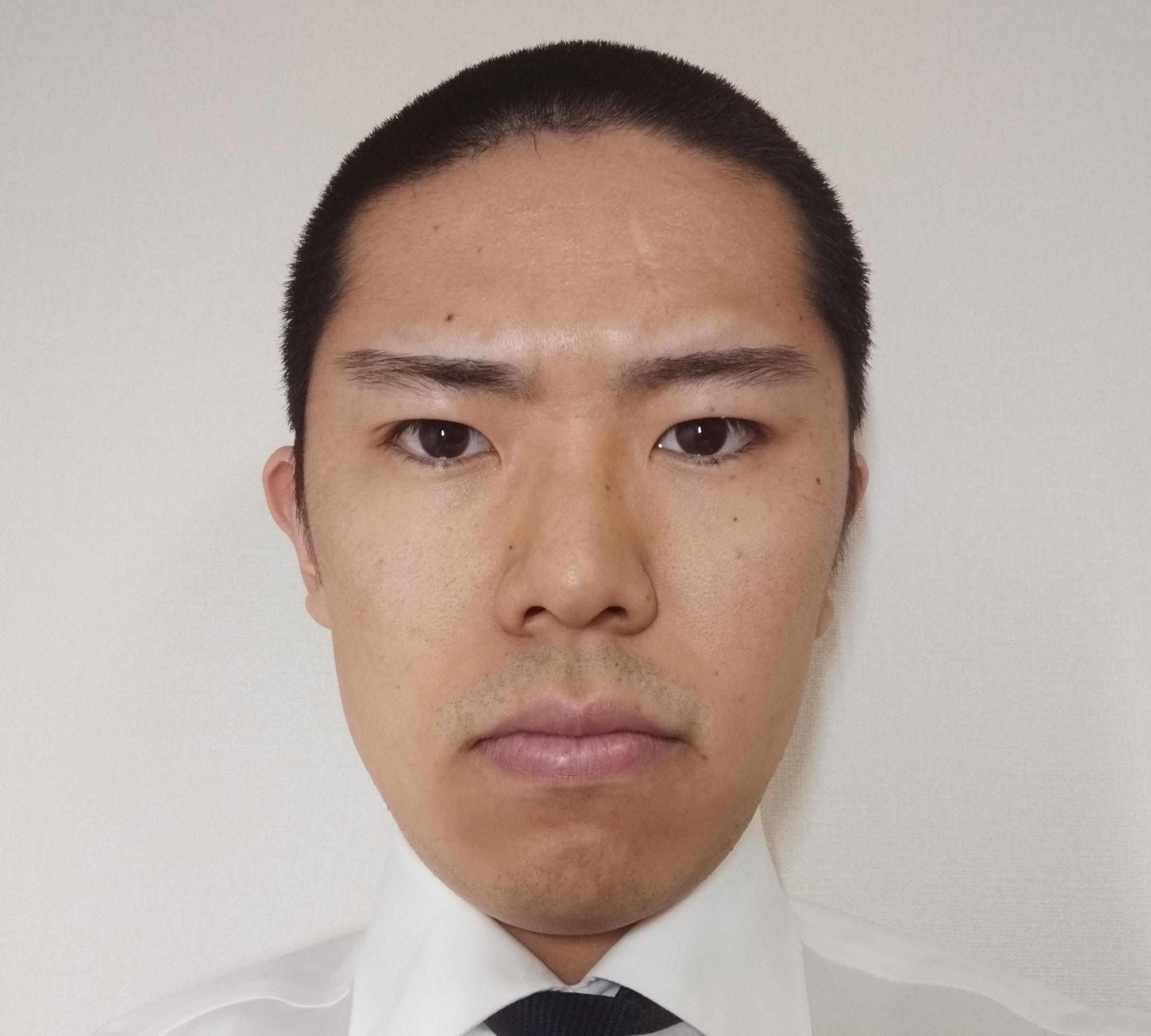
Haruki Niwa
Researcher
I left my postgraduate studies at Aichi Prefectural University in 2022 and joined Araya. I aim to reduce mental suffering using technology.
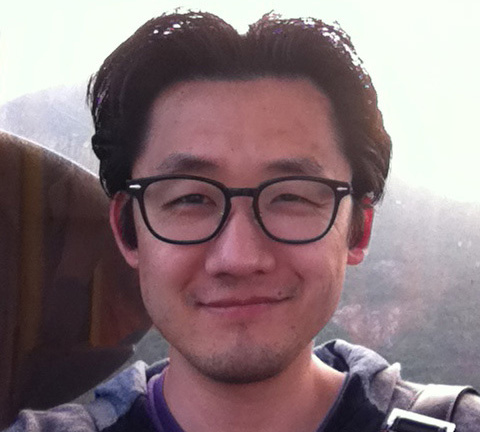
Dan Lee
Senior Researcher
Dan is a researcher at Araya. He received his PhD in Psychology at the University of Toronto, and his BASc in Computer Engineering at the University of Waterloo. He is interested in emotions and value, their representations, and their function in intelligence.