Members
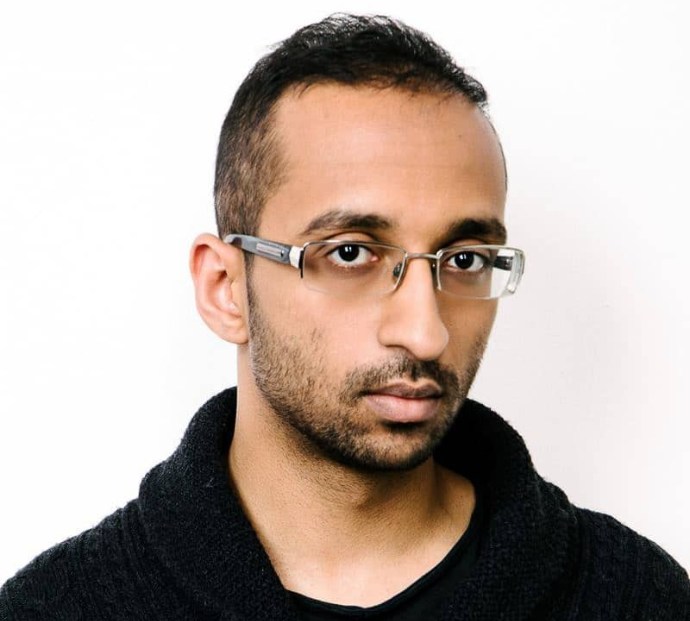
Kai Arulkumaran, Ph.D.
Research Team Lead
Kai is a Research Team Leader at Araya and Visiting Researcher at Imperial College London. He received his B.A. in Computer Science at the University of Cambridge in 2012 and his Ph.D. in Bioengineering at Imperial College London in 2020. He has previously worked at DeepMind, Microsoft Research, Facebook AI Research, Twitter and NNAISENSE. His research interests are deep learning, reinforcement learning, evolutionary computation and theoretical neuroscience.
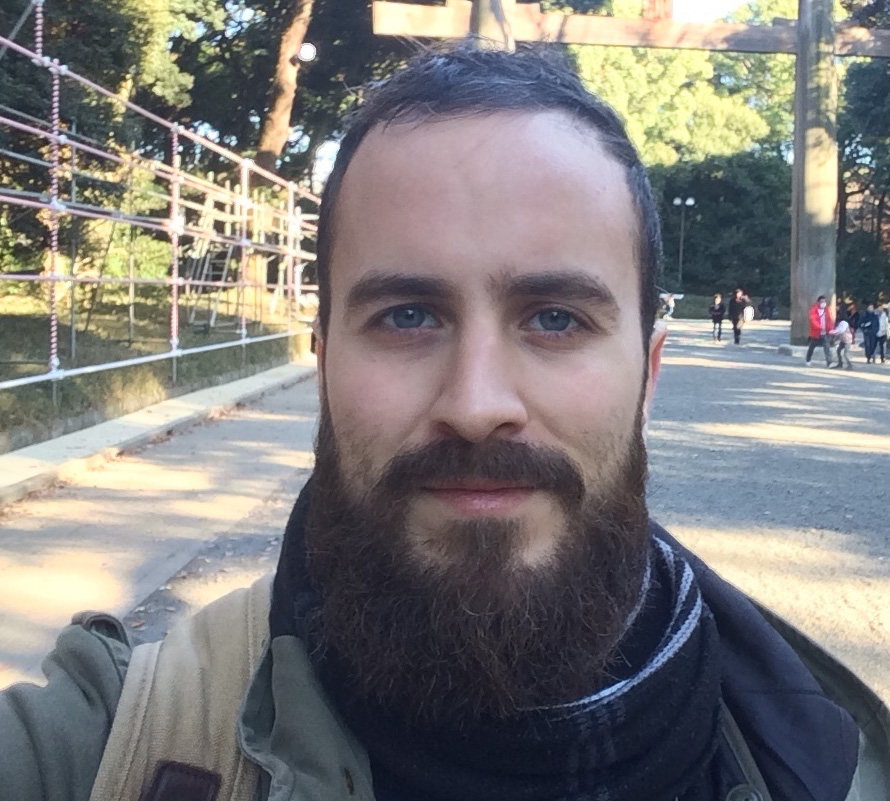
Manuel Baltieri, Ph.D.
Chief Researcher
Manuel is a Chief Researcher at Araya and a Visiting Researcher at the University of Sussex. After graduating with a B.Eng. in Computer Engineering and Business Administration at the University of Trento, he received an M.Sc. in Evolutionary and Adaptive Systems and a Ph.D. in Computer Science and AI, both from the University of Sussex. Following that, he was awarded a JSPS/Royal Society postdoctoral fellowship, and worked in the Lab for Neural Computation and Adaptation at RIKEN CBS with Taro Toyoizumi, until he joined Araya at the end of 2021. His research interests include artificial intelligence and artificial life, theories of agency and individuality, origins of life, embodied cognition and decision making.
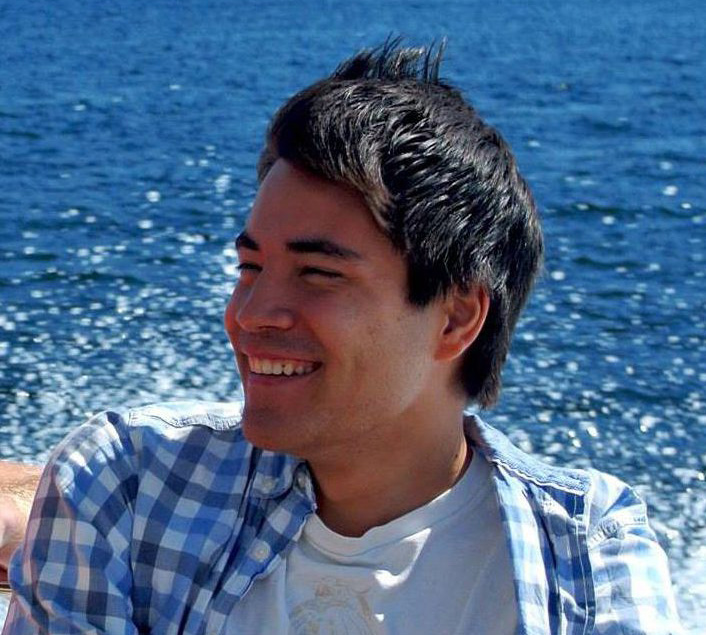
Dan Ogawa Lillrank
Chief Researcher
Dan is a Chief Researcher at Araya. He received his B.A. in Engineering Physics at Royal Institute of Technology Sweden in 2015, and his M.S. in Robotics at 2018. He has previously worked at Telexistence Inc, and as a Researcher at AIST. His research interests are mainly in robotics learning, the intersection of machine learning & robotics.
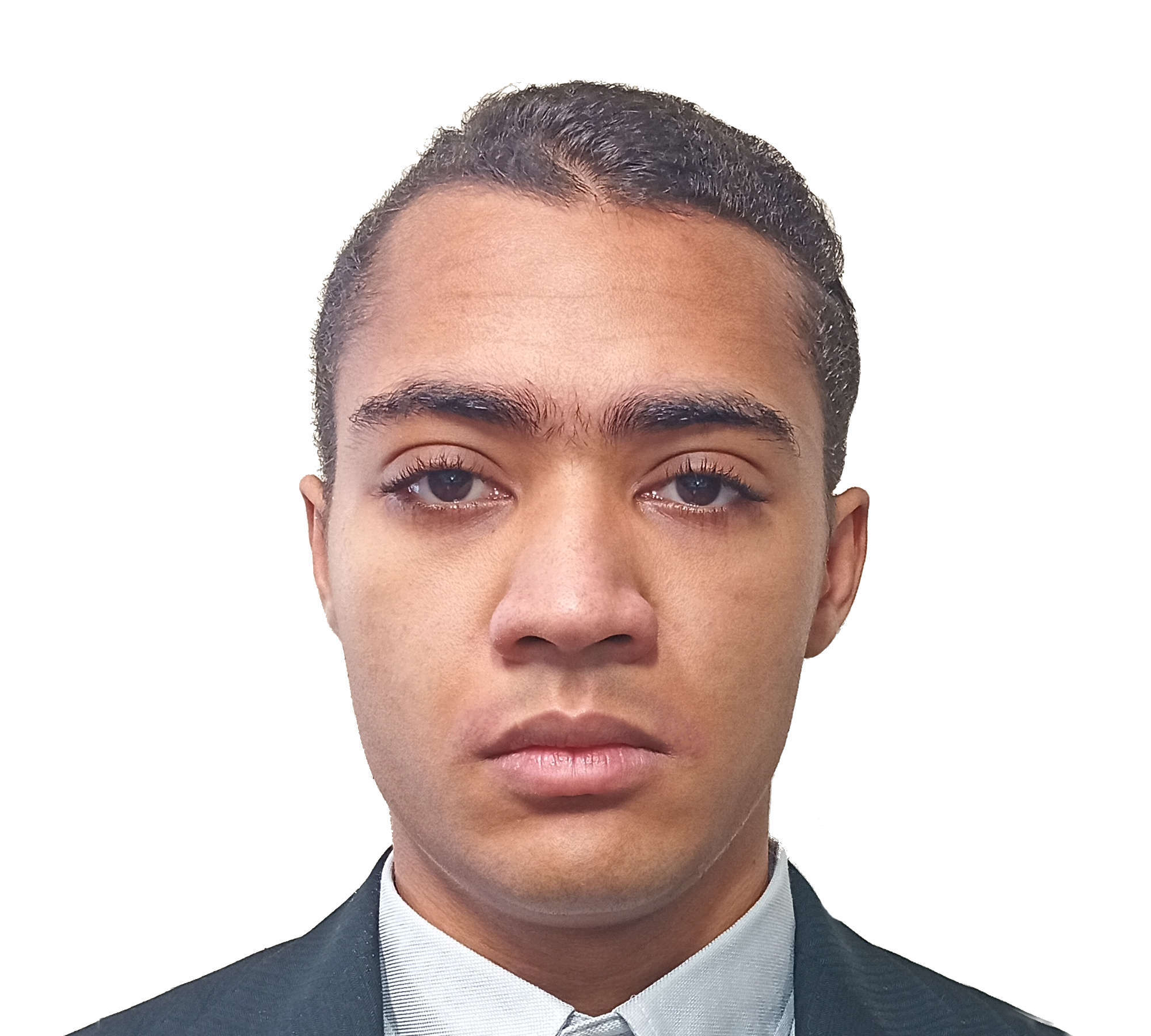
Rousslan Dossa, Ph.D.
Senior Researcher
Rousslan is a Senior Researcher at Araya. He received his Ph.D. from Kobe University in 2023. His research interests span over the topics of deep reinforcement learning with an emphasis on self-supervised learning, human cognition-inspired decision-making, neuroscience, and evolutionary computing.
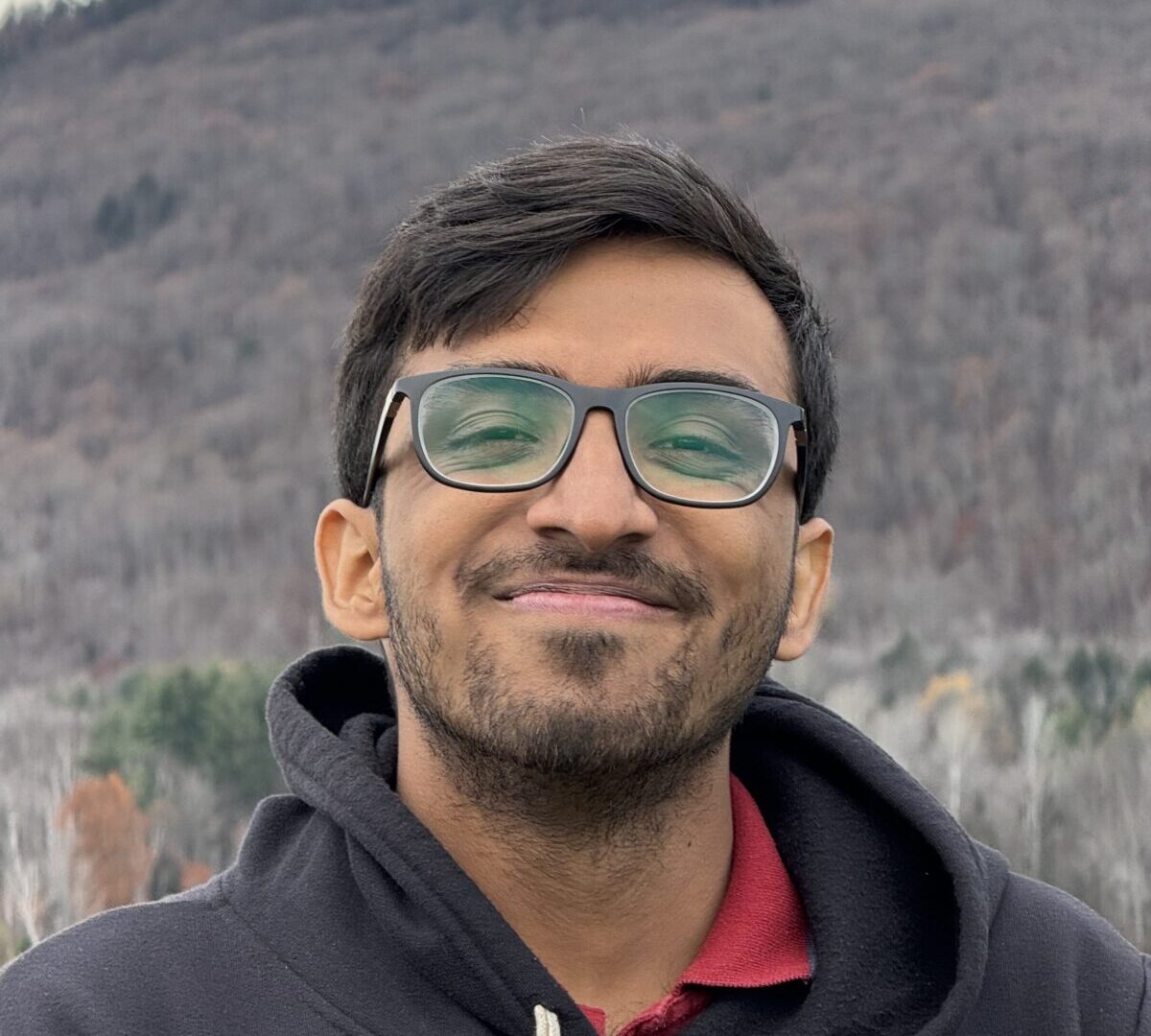
Shivakanth Sujit
Senior Researcher
Shivakanth is a Senior Researcher at Araya. He received his M.Sc. in 2023 from Mila Quebec working with Prof Samira Ebrahimi Kahou. He is interested in deep reinforcement learning for robotics and LLMs. Before joining Mila he completed his undergraduate at NIT Trichy, India in Control Engineering, and this background drives his research in combining the insights from control theory and RL for building agents that can safely interact in the real world.
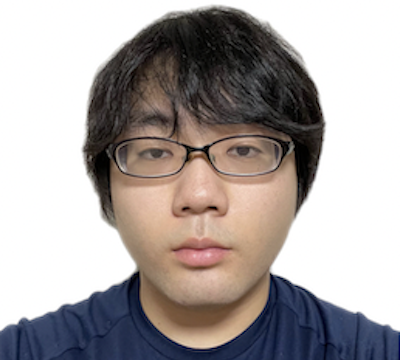
Shogo Akiyama
Senior Researcher
Shogo is a Senior Researcher at Araya. He received his B.S. in Computer Science in 2019. He has previously worked as an AI and web application engineer. His research interests are reinforcement learning and natural language processing.
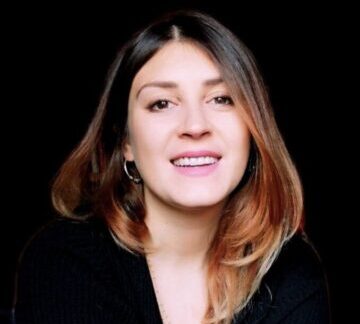
Marina Di Vincenzo
Senior Researcher
Marina is a Senior Researcher at Araya. She received her B.A. in Psychology at the University of Urbino “Carlo Bo” in 2017; her M.S. in Neuroscience and Psychological Rehabilitation in 2021 at the University “La Sapienza” of Rome; and a Diploma in Artificial Intelligence, the same year, at the Institute of Sciences and Technologies of Cognition of the National Research Council (ISTC-CNR).
Her research focuses on User-Centered Design in Assistive Neurotechnology.
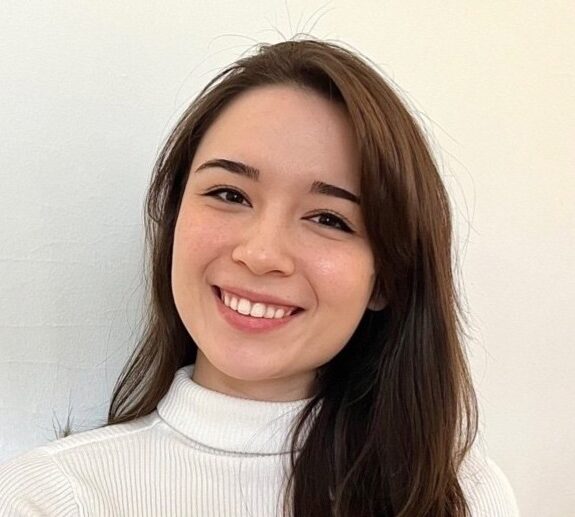
Hannah Kodama Douglas
Senior Researcher
Hannah is a Senior Researcher at Araya. She received her B.S. in Statistics at Carnegie Mellon University in 2020 before completing her postbac at the National Institutes of Health in the Unit of Neural Computation and Behavior. She then received her M.S. in Computational Neuroscience from Princeton University in 2024. She's interested in exploring ways to apply insights from neuroscience and machine learning to develop practical brain-machine interfaces.
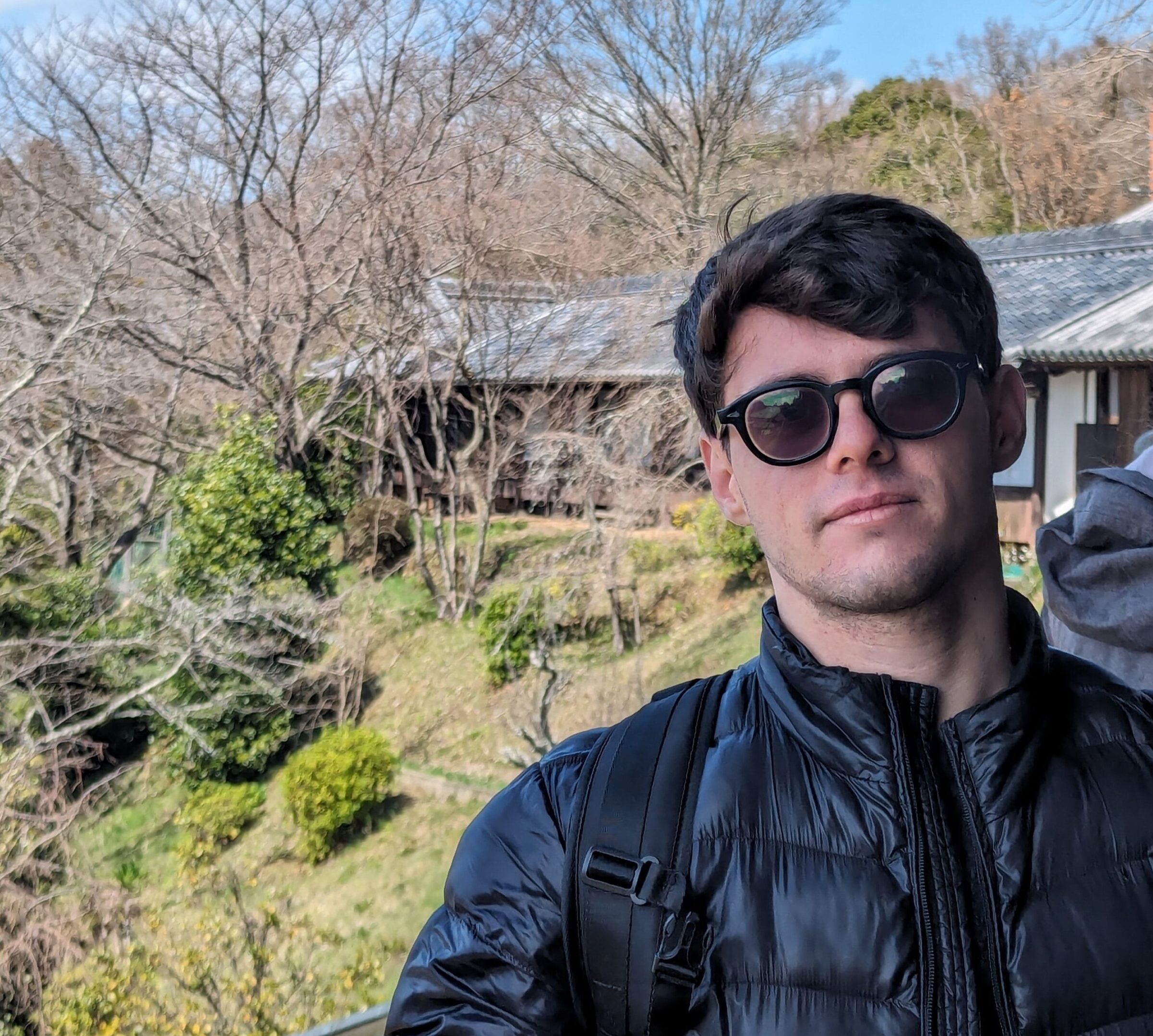
Luca Nunziante
Senior Researcher
Luca is a Senior Researcher at Araya. After receiving his bachelor's degree in Electronic and Computer Engineering at the University of Campania Luigi Vanvitelli, he completed his M.Sc. in Artificial Intelligence and Robotics at La Sapienza University of Rome in 2024. During his master he visited the Space Robotics Laboratory at Tohoku University, Japan. His research interest are robot control, artificial intelligence, and the intersection of the two.