Members
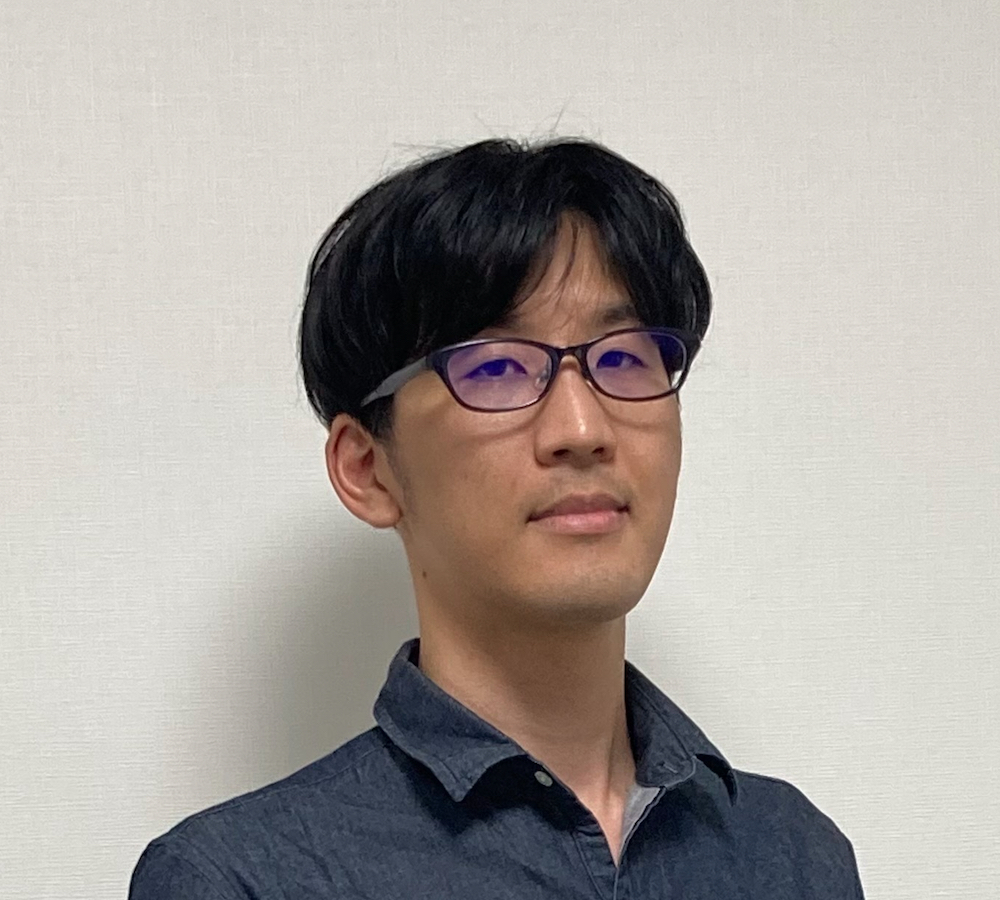
Ippei Fujisawa,Ph.D.
Research Team Lead
Ippei is a Research Team Leader at Araya. He recieved his Ph.D in Theoretical Particle Physics at Hokkaido University in 2016. His research interests include deep learning, computer vision and meta learning.
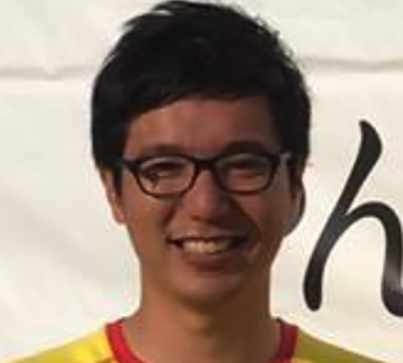
Hiro Hamada, Ph.D.
Research Team Lead
Hiro is a Research Team Lead at Araya. He received his Ph.D in systems neuroscience at Okinawa Institute of Science and Technology (OIST) in 2019. His research interests are cognitive neuroscience, computational neuroscience and phenomenology of consciousness.
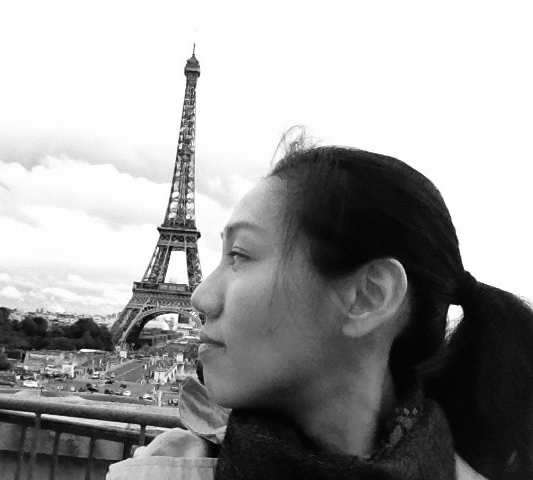
Yi-Chen Lin, Ph.D.
Product Development Lead
Yi-Chen is a Product Development Lead at Araya. She received her Ph.D. at National Yang-Ming University in 2019. Her research interest are cognitive neuroscience and neuroimaging.